The Ultimate Guide to Data Annotation Tools
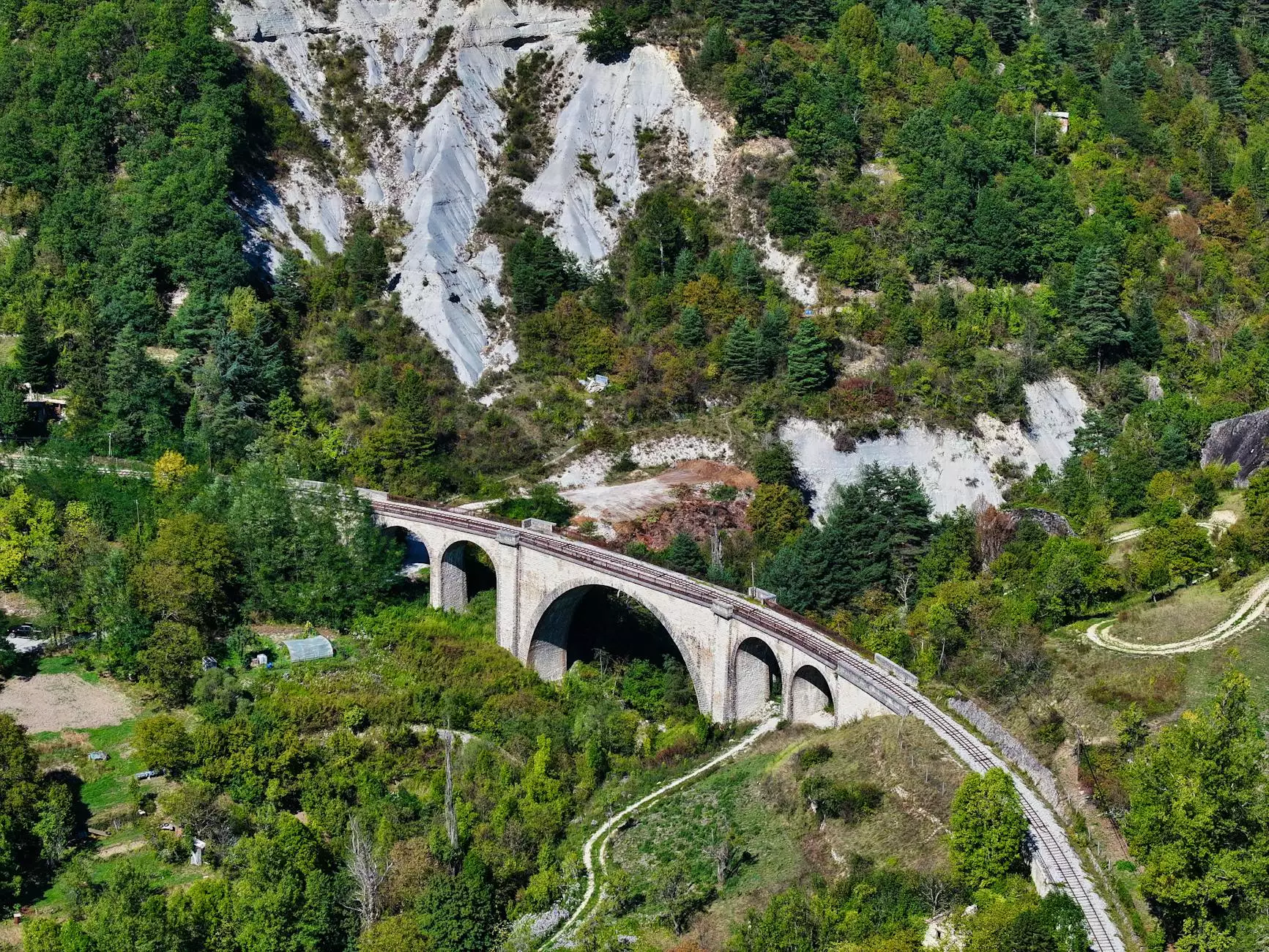
Data annotation tools are becoming increasingly vital in the realm of software development, especially as businesses harness the power of artificial intelligence (AI) and machine learning (ML). In today's fast-paced digital landscape, accurate data labeling shapes the foundation of reliable and efficient AI systems. This comprehensive guide will delve into the importance of data annotation tools, their applications, and how utilizing them effectively can transform your business operations.
What is Data Annotation?
Data annotation is the process of labeling data, which can come in various forms, including images, audio files, and text. This task is crucial for training machine learning models, as these models require large amounts of classified data to learn from. By using data annotation tools, businesses can streamline this process, ensuring accuracy and precision while significantly reducing the time needed for data preparation.
Types of Data Annotation
- Image Annotation: Involves marking specific areas of images to identify objects, actions, or features.
- Text Annotation: Entails labeling parts of written content to provide context, such as sentiment or intent.
- Audio Annotation: Involves transcribing audio files and labeling different segments based on tone, emotion, or specific commands.
- Video Annotation: The process of tagging frames in a video to identify objects or actions over time.
Why is Data Annotation Important for Businesses?
In the digital age, data is referred to as the new oil; it powers most businesses and drives decision-making. Here’s why employing data annotation tools is essential:
1. Enhancing Machine Learning Models
Machine learning models are only as good as the data they are trained on. Well-annotated data leads to higher accuracy and reliability of AI systems. Businesses leveraging accurate annotated data can see improved performance in applications ranging from natural language processing to image recognition.
2. Cost Efficiency
Manually annotating data can be time-consuming and expensive. By utilizing automated data annotation tools, businesses can save resources, focusing their human workforce on more complex tasks that require critical thinking and creativity.
3. Scalability
As your business grows, so does your data. An efficient data annotation tool can scale with your organization, continuously processing and labeling new incoming data without compromising on quality.
Key Features of Effective Data Annotation Tools
When selecting a data annotation tool for your business, consider the following features to ensure you are making a wise investment:
- User-Friendly Interface: The tool should be intuitive and easy to navigate for all users, regardless of technical expertise.
- Versatility: It should support various data types, including images, text, audio, and video, for diversified use cases.
- Collaboration Features: Ability for multiple users to work together seamlessly, providing feedback and results in real time.
- Automation Capabilities: Some tasks can be automated to speed up the data annotation process.
- Quality Control Mechanisms: Tools should have built-in features for ensuring the accuracy and consistency of annotations.
Popular Data Annotation Tools on the Market
Choosing the right data annotation tool can make all the difference in your business. Here are some of the top tools widely recognized in the industry:
- Labelbox: A comprehensive platform that offers image, video, text, and audio annotation, all with collaboration and quality control features.
- SuperAnnotate: Focused on image and video annotations, SuperAnnotate provides a user-friendly interface and automation capabilities.
- VGG Image Annotator: A simple yet effective tool for annotating images, primarily used for academic purposes.
- DataRobot: Integrates data annotation with machine learning operations, making it ideal for organizations looking to incorporate AI solutions.
Integrating Data Annotation Tools into Your Workflow
To fully benefit from data annotation tools, businesses need to seamlessly integrate them into their existing workflows. Here are some strategies for successful integration:
1. Define Objectives
Before implementing an annotation tool, outline your goals. What type of data will you be annotating? What specific outcomes do you expect? Clear objectives will guide your selection process.
2. Train Your Team
Ensure that your team is well-trained in utilizing the selected data annotation tool. A little investment in training can pay off significantly in terms of productivity.
3. Implement Quality Control Processes
Create a framework for reviewing and verifying the annotations produced. This step is crucial in maintaining the accuracy required for machine learning algorithms.
Real-World Applications of Data Annotation
Data annotation tools have a plethora of applications across different industries. Here are a few examples:
1. Healthcare
In the healthcare sector, data annotation is used for medical imaging, where AI models can help in diagnosing diseases by identifying abnormalities in scans.
2. Automotive
In autonomous driving technology, annotated data, particularly from videos, is essential for training AI systems to recognize traffic signs and human pedestrians.
3. E-commerce
Data annotation helps personalize customer experiences by training recommendation engines to better understand user behavior and preferences.
The Future of Data Annotation Tools
The landscape of data annotation tools is rapidly evolving. With advancements in AI and technology, we can expect the following trends:
1. Increased Automation
As NLP and computer vision technologies advance, expect a shift towards automated annotation processes, making it easier and faster to label massive datasets.
2. Enhanced Collaboration Features
With remote work becoming the norm, tools are likely to enhance their collaboration capabilities, allowing teams from different locations to work together seamlessly.
3. Focus on Privacy and Ethics
As data privacy laws become stringent, data annotation tools will need to incorporate features that safeguard sensitive information, ensuring compliance with regulations.
Conclusion
Investing in effective data annotation tools is crucial for businesses aiming to leverage the power of artificial intelligence and machine learning successfully. These tools not only streamline the data labeling process but also enhance the accuracy and efficiency of machine learning models. As we move forward, companies that adopt advanced data annotation strategies will gain a significant competitive edge in their respective markets.
By understanding the types, benefits, and applications of data annotation tools, organizations can make informed decisions that contribute to their long-term success.